How User Research Can Unlock The Full Potential of AI Tools

In the past year, Artificial Intelligence (AI) has undergone a remarkable evolution that has significantly impacted various industries worldwide.
We’ve witnessed the development of language processing tools such as OpenAI’s ChatGPT-3 and Google’s Bard, both of which have shown unmatched capabilities in generating human-like text, including writing poetry, summarising long articles and answering complex questions. Microsoft’s Power Fx is now being used to build custom business applications with ease. In the medical field, AI-powered diagnostic tools have been developed to assist in the detection and prediction of chronic conditions. DALL-E2, with its ability to create unique and imaginative images from text prompts has shown potential to transform creative industries, e-commerce and education. Finally, AI-powered chatbots such as IBM Watson Assistant have become increasingly prevalent in customer service, allowing for more efficient and personalised interactions with customers.
As these AI tools continue to evolve and expand swiftly and impressively, with exciting possibilities for improving our lives and industries, it’s become not only necessary but crucial to ask the question:
What role can we as UX researchers play in the development of AI?
AI is a wide-ranging term that encompasses various tools and supporting technologies. Some of these tools are more technical and specialised than others while some are designed for consumer usage.
This can be demonstrated by sorting some typical examples of AI-powered tools into categories based on their method of operation.
- Recommendation engines: These algorithms learn from user data to suggest relevant content, products, or services. Everyday examples include e-commerce platforms like Amazon.com, streaming platforms like Netflix, or social media feeds like Twitter.
- Chatbots and virtual assistants: These tools process human language and simulate conversation for various tasks. Everyday examples include Alexa, Siri, and customer support chatbots.
- Computer vision: These tools analyse images and videos to detect and recognise objects and faces. Autonomous vehicles, augmented reality and image classification are some of its advanced use cases. Such tools can also be commonly found on social media, photo sharing apps, and home security devices.
- Generative AI: These tools generate content based on a ‘prompt’ or input. Examples include art generators like DALL-E 2, text models like ChatGPT, and hybrid tools like Google Duplex.
Since it is quite common for non-expert consumers of AI to be unaware of the form of AI that is powering a specific tool, three key opportunities stand out as areas where UX researchers can supplement the user experience of AI.
1 — Help users form appropriate mental models

As Cooper, A. et al describe in their book About Face, “from a moviegoer’s point of view, it is easy to forget the nuance of sprocket holes and light interrupters while watching an absorbing drama.” It is common for viewers, in fact, to have little idea about how a projector even works, or how it differs from the way a television works. If questioned, viewers might typically explain that the projector simply throws a moving picture on to the screen. This is an example of a mental model, or conceptual model. It is a presumed explanation of how something works.
User interfaces, also known as represented models (since they act as the face a product shows to the world), that are consistent with users’ mental models are commonly considered vastly superior to those that are merely reflections of the products’ implementation model, that is, its complex technical mechanism. This is based on the logic that when users can easily understand how to use a product, they are more likely to engage with it frequently and use more of its features. Therefore, as long as television viewers intuitively know how to use a TV, they will remain satisfied - albeit not particularly understanding the underlying technology.
When it comes to AI tools, however, there is a further requirement.
In the case of AI, more important than knowing what a tool can and can’t do is having some approximate understanding of how it does things and why it can’t do others.
These systems are extraordinarily powerful and can increasingly do everything a human can do — or better and faster. But their enhanced capabilities also give them the potential to deceive and mislead us — at least while AI is still in its infancy and challenges of AI discrimination and bias exist. And a lack of awareness of their underlying mechanism means that it isn’t far-fetched for novice users to believe AI systems can’t make mistakes at all.
Eliminating the risk from such misconceptions would require designing AI systems whose internals are understood by end users and whose goals we are collectively able to shape to be safe ones.
ChatGPT, for example, has been trained on millions of pieces of writing to learn when words are most likely to follow each other in a given context — a kind of ‘autocomplete on steroids’. As users begin to understand that AI tools are trained to derive relationships between variables in large datasets and have no abstract understanding of the underlying concepts, they will feel equipped to avoid undesirable outcomes and achieve more desirable ones.
UX research can help elicit current mental models, provide clues to users about the mechanism of AI tools, and test the models conveyed by new design concepts.
2 — Help users trust output to be safe and reliable

Although we tend to view AI as an impartial tool, it is, in reality, heavily influenced by the biases of its creators and the data it is trained on. If these biases are not addressed, we run the risk of eroding confidence in AI’s outputs and perpetuating unjust social dynamics.
For instance, facial recognition technology may be less precise for individuals with darker skin tones, resulting in potential bias in law enforcement. Furthermore, there is a history of language models being removed from public release after producing offensive responses with harmful stereotypes.
However, it’s not just about bias; AI tools are also prone to ‘hallucinate’, or make erroneous assertions with certainty. This can manifest in many ways, including non-existent references or erroneous calculations.
Both bias and hallucinations can have a significant impact on the ability of AI tools to be valuable in critical applications such as medicine, law, education, or research.
One potential solution is the principle of Explainable AI. Another strategy, as recommended by Gavin Lew and Bob Schumacher of Bold Insight, is to have individuals trained in the nuances of technology and social science, such as UX researchers, collect the training data in a clear and principled manner.
By providing transparency into the inner workings of AI systems, we as UX researchers can instil confidence in their outputs and promote responsible use.
3 — Help users collaborate with the tool
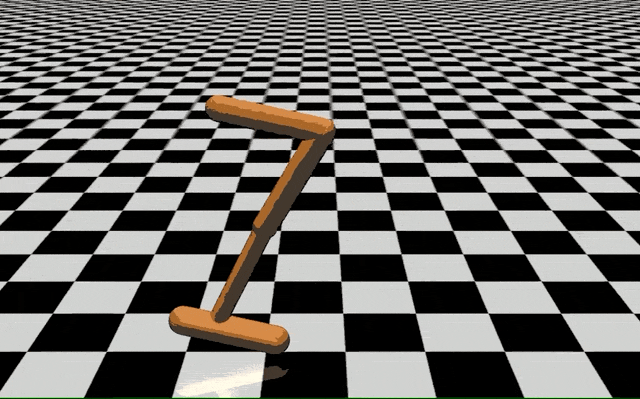
When humans collaborate with AI systems, results are often better than what either humans or AI systems could potentially achieve alone.
For example, in a study involving an online protein-folding simulation called Foldit, the application presented a challenge that had stumped scientists for over a decade. This problem, however, was quickly solved by a group of Foldit players working collaboratively with an AI system. The AI system provided players with potential folding solutions, and the players used their human intuition to select the best option. This combined approach resulted in a solution that demonstrated the potential for these technologies to work together to solve complex scientific problems more efficiently than either could do alone.
It is such collaborative abilities that can differentiate job candidates in the market as well, which is good news for individuals concerned about job security. While the initial output of an AI tool may not always be optimal, however, collaborating with human intelligence can enhance its results. This is why many successful recommendation engines take user feedback into consideration, in addition to behavioural data. Similarly, when generating artwork with AI tools, users often refine prompts through iterative processes to enhance the final image.
UX research can identify effective approaches to promote this partnership. The crucial aspect is to learn how to work efficiently with AI, rather than solely depending on it or fearing it.
Summary
In summary, AI tools — whether recommendation engines, chatbots, computer vision, or generative AI — offer incredible potential. But without proper UX research, that value will remain limited.
Improvements in these areas may help overcome user resistance and other barriers to adoption. And they may also help users achieve better results from tools that would be otherwise technically equivalent. Ultimately, AI tools with the best user experience will have an edge in a highly competitive space.